
Companies require systems for diverse data applications including SQL analytics, real-time monitoring, data science, and machine learning. The need for a flexible, high-performance system hasn’t abated. For these reasons, many of the promises of the data lakes have not materialized, and in many cases leading to a loss of many of the benefits of data warehouses. While suitable for storing data, data lakes lack some critical features: they do not support transactions, they do not enforce data quality, and their lack of consistency / isolation makes it almost impossible to mix appends and reads, and batch and streaming jobs. About a decade ago companies began building data lakes - repositories for raw data in a variety of formats. Data warehouses are not suited for many of these use cases, and they are certainly not the most cost efficient.Īs companies began to collect large amounts of data from many different sources, architects began envisioning a single system to house data for many different analytic products and workloads. But while warehouses were great for structured data, a lot of modern enterprises have to deal with unstructured data, semi-structured data, and data with high variety, velocity, and volume. Since its inception in the late 1980s, data warehouse technology continued to evolve and MPP architectures led to systems that were able to handle larger data sizes. In this post we describe this new architecture and its advantages over previous approaches.ĭata warehouses have a long history in decision support and business intelligence applications.
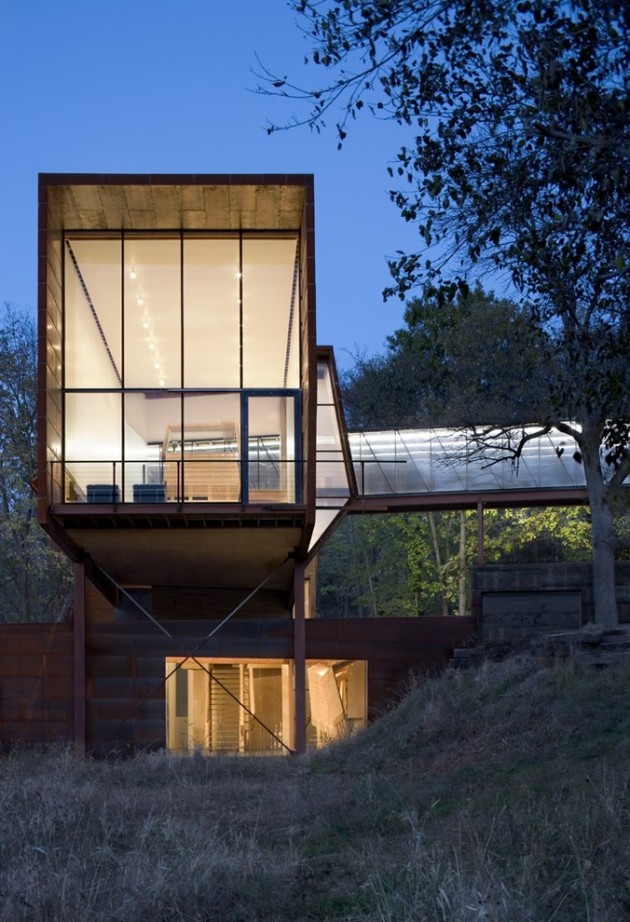

Over the past few years at Databricks, we've seen a new data management architecture that emerged independently across many customers and use cases: the lakehouse.
